La «predicción de series temporales» se refiere a la tarea de prever valores futuros en una secuencia de datos organizada en función del tiempo. En otras palabras, implica hacer estimaciones o pronósticos sobre cómo evolucionarán los datos en el futuro, basándose en patrones y tendencias observadas en el pasado.
Estos modelos pueden ser muy efectivos para tareas como predecir valores futuros en el mercado de valores, el clima, la demanda de productos, predicción del tráfico y mucho más.
import tensorflow as tf
import numpy as np
import matplotlib.pyplot as plt
# Datos de ejemplo: serie temporal univariada (ajusta esto con tus datos)
series_temporales = np.sin(0.1 * np.arange(200)) + np.random.randn(200) * 0.1
# Función para crear secuencias de datos y sus etiquetas
def create_sequences(data, window_size):
sequences = []
labels = []
for i in range(len(data) - window_size):
sequences.append(data[i:i+window_size])
labels.append(data[i+window_size])
return np.array(sequences), np.array(labels)
# Dividir los datos en conjuntos de entrenamiento y prueba
window_size = 10
X, y = create_sequences(series_temporales, window_size)
split = int(0.8 * len(X))
X_train, X_test, y_train, y_test = X[:split], X[split:], y[:split], y[split:]
# Crear el modelo de la RNN
model = tf.keras.Sequential([
tf.keras.layers.SimpleRNN(32, input_shape=(window_size, 1)),
tf.keras.layers.Dense(1)
])
# Compilar el modelo
model.compile(optimizer='adam', loss='mse') # Mean Squared Error
# Entrenar el modelo
model.fit(X_train, y_train, epochs=50, verbose=0)
# Evaluar el modelo
test_loss = model.evaluate(X_test, y_test)
print(f'Pérdida en el conjunto de prueba: {test_loss:.4f}')
# Predecir valores futuros
future = 50 # Número de puntos en el futuro para predecir
last_sequence = X[-1] # Última secuencia de la serie temporal
predicted = []
for _ in range(future):
next_value = model.predict(last_sequence.reshape(1, window_size, 1))
predicted.append(next_value[0, 0])
last_sequence = np.roll(last_sequence, shift=-1)
last_sequence[-1] = next_value[0, 0]
# Visualizar la serie temporal original y las predicciones
plt.plot(np.arange(len(series_temporales)), series_temporales, label='Serie Temporal Original')
plt.plot(np.arange(len(series_temporales), len(series_temporales) + future), predicted, label='Predicciones Futuras')
plt.legend()
plt.show()
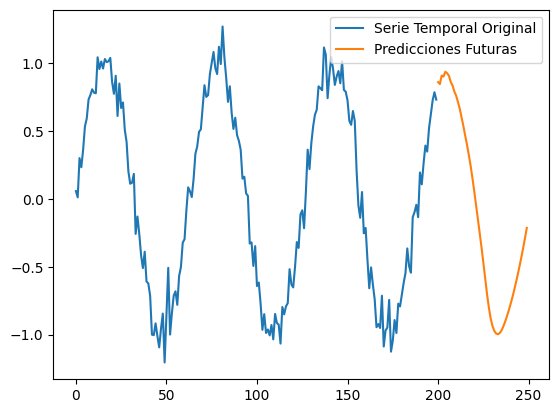
Explicación del código:
- Generación de Datos de Serie Temporal:
- En el código, se crea una serie temporal univariada de ejemplo. Esta serie temporal se genera como una función seno con ruido aleatorio. Puedes modificar esta parte del código para utilizar tus propios datos de serie temporal. La serie temporal de ejemplo se almacena en la variable
series_temporales
.
- En el código, se crea una serie temporal univariada de ejemplo. Esta serie temporal se genera como una función seno con ruido aleatorio. Puedes modificar esta parte del código para utilizar tus propios datos de serie temporal. La serie temporal de ejemplo se almacena en la variable
- Creación de Secuencias y Etiquetas:
- Para entrenar una RNN, es necesario crear secuencias de datos junto con sus etiquetas correspondientes. El código define una función llamada
create_sequences
que toma la serie temporal y una ventana deslizante y crea secuencias de datos (ventanas) junto con las etiquetas correspondientes. Estas secuencias y etiquetas se almacenan en las variablesX
yy
.
- Para entrenar una RNN, es necesario crear secuencias de datos junto con sus etiquetas correspondientes. El código define una función llamada
- División de Datos en Conjuntos de Entrenamiento y Prueba:
- Luego, el código divide los datos en conjuntos de entrenamiento (
X_train
yy_train
) y prueba (X_test
yy_test
) para poder evaluar el modelo de manera efectiva. En este ejemplo, se utiliza el 80% de los datos como entrenamiento y el 20% restante como prueba.
- Luego, el código divide los datos en conjuntos de entrenamiento (
- Construcción del Modelo RNN:
- El modelo de RNN se construye utilizando la biblioteca Keras de TensorFlow. En este caso, se utiliza un modelo secuencial de Keras. El modelo consta de una capa RNN (una capa SimpleRNN) y una capa de salida densa. La capa RNN se utiliza para aprender patrones temporales en los datos. El número de unidades en la capa RNN se establece en 32.
- Compilación del Modelo:
- El modelo se compila configurando el optimizador y la función de pérdida. En este ejemplo, se utiliza el optimizador ‘adam’ y la función de pérdida ‘mse’ (Mean Squared Error), que es comúnmente utilizada en problemas de regresión. El modelo se compila para minimizar la pérdida cuadrática media entre las predicciones y las etiquetas reales.
- Entrenamiento del Modelo:
- El modelo se entrena en los datos de entrenamiento (
X_train
yy_train
) durante un número específico de épocas (en este caso, 50). Durante el entrenamiento, el modelo ajusta sus pesos para aprender los patrones de la serie temporal.
- El modelo se entrena en los datos de entrenamiento (
- Evaluación del Modelo:
- Después del entrenamiento, el modelo se evalúa en el conjunto de prueba (
X_test
yy_test
) para medir su capacidad para hacer predicciones precisas. La pérdida en el conjunto de prueba se calcula y se muestra en la consola.
- Después del entrenamiento, el modelo se evalúa en el conjunto de prueba (
- Predicción de Valores Futuros:
- Finalmente, el modelo se utiliza para hacer predicciones de valores futuros en la serie temporal. El código predice los próximos valores en la serie y los almacena en la variable
predicted
. Estas predicciones se generan a partir de la última secuencia de datos en la serie temporal.
- Finalmente, el modelo se utiliza para hacer predicciones de valores futuros en la serie temporal. El código predice los próximos valores en la serie y los almacena en la variable
- Visualización de Resultados:
- Para visualizar los resultados, el código utiliza Matplotlib. La serie temporal original y las predicciones se trazan en un gráfico para permitir una comparación visual. Esto muestra cómo el modelo se desempeña en la tarea de predecir valores futuros en la serie temporal.
Noodlemagazine I really like reading through a post that can make men and women think. Also, thank you for allowing me to comment!
obviously like your website but you need to test the spelling on quite a few of your posts Several of them are rife with spelling problems and I to find it very troublesome to inform the reality on the other hand Ill certainly come back again
Somebody essentially lend a hand to make significantly posts I might state That is the very first time I frequented your web page and up to now I surprised with the research you made to create this particular put up amazing Excellent job
Noodlemagazine Hi there to all, for the reason that I am genuinely keen of reading this website’s post to be updated on a regular basis. It carries pleasant stuff.
I loved as much as you’ll receive carried out right here. The sketch is attractive, your authored material stylish. nonetheless, you command get bought an nervousness over that you wish be delivering the following. unwell unquestionably come more formerly again as exactly the same nearly a lot often inside case you shield this hike.
Can you be more specific about the content of your article? After reading it, I still have some doubts. Hope you can help me.
I loved as much as you’ll receive carried out right here. The sketch is tasteful, your authored material stylish.
NY weekly This was beautiful Admin. Thank you for your reflections.
Your point of view caught my eye and was very interesting. Thanks. I have a question for you.
I don’t think the title of your article matches the content lol. Just kidding, mainly because I had some doubts after reading the article.
I just wanted to say that your article is remarkable. The clarity and depth of your knowledge are truly refreshing. May I subscribe to keep up with your future posts? Keep up the fantastic work!
Mangaclash very informative articles or reviews at this time.
Thinker Pedia I really like reading through a post that can make men and women think. Also, thank you for allowing me to comment!
Keep up the fantastic work! Kalorifer Sobası odun, kömür, pelet gibi yakıtlarla çalışan ve ısıtma işlevi gören bir soba türüdür. Kalorifer Sobası içindeki yakıtın yanmasıyla oluşan ısıyı doğrudan çevresine yayar ve aynı zamanda suyun ısınmasını sağlar.
Blue Techker Great information shared.. really enjoyed reading this post thank you author for sharing this post .. appreciated
Fran Candelera Nice post. I learn something totally new and challenging on websites
Insanont There is definately a lot to find out about this subject. I like all the points you made
Mating Press I like the efforts you have put in this, regards for all the great content.
Technoob I very delighted to find this internet site on bing, just what I was searching for as well saved to fav
Its like you read my mind You appear to know so much about this like you wrote the book in it or something I think that you can do with a few pics to drive the message home a little bit but other than that this is fantastic blog A great read Ill certainly be back
Pink Withney This was beautiful Admin. Thank you for your reflections.
Hi my family member I want to say that this post is awesome nice written and come with approximately all significant infos I would like to peer extra posts like this
I do not even know how I ended up here but I thought this post was great I dont know who you are but definitely youre going to a famous blogger if you arent already Cheers
Tech to Trick This is really interesting, You’re a very skilled blogger. I’ve joined your feed and look forward to seeking more of your magnificent post. Also, I’ve shared your site in my social networks!
Hi i think that i saw you visited my web site thus i came to Return the favore Im attempting to find things to enhance my siteI suppose its ok to use a few of your ideas
Real Estate I like the efforts you have put in this, regards for all the great content.
Mygreat learning I’m often to blogging and i really appreciate your content. The article has actually peaks my interest. I’m going to bookmark your web site and maintain checking for brand spanking new information.
Real Estate naturally like your web site however you need to take a look at the spelling on several of your posts. A number of them are rife with spelling problems and I find it very bothersome to tell the truth on the other hand I will surely come again again.
Real Estate naturally like your web site however you need to take a look at the spelling on several of your posts. A number of them are rife with spelling problems and I find it very bothersome to tell the truth on the other hand I will surely come again again.
Obrigado, estou procurando informações sobre esse tópico há algum tempo e a sua é a melhor que descobri até agora. Mas e em relação aos resultados financeiros? Você tem certeza sobre o fornecimento
olá, gosto muito da sua escrita, tanto que mantemos uma correspondência extra sobre sua postagem na AOL. Preciso de um especialista neste espaço para desvendar meu problema. Talvez seja você. Estou ansioso para vê-lo
Vitazen Keto Gummies Good post! We will be linking to this particularly great post on our site. Keep up the great writing
Excellent blog here Also your website loads up very fast What web host are you using Can I get your affiliate link to your host I wish my web site loaded up as quickly as yours lol
Temp mail I am truly thankful to the owner of this web site who has shared this fantastic piece of writing at at this place.
Your words have a way of touching hearts and inspiring minds Thank you for using your platform to spread love and positivity
Fantastic site Lots of helpful information here I am sending it to some friends ans additionally sharing in delicious And of course thanks for your effort
Excellent blog here Also your website loads up very fast What web host are you using Can I get your affiliate link to your host I wish my web site loaded up as quickly as yours lol
Fantastic site Lots of helpful information here I am sending it to some friends ans additionally sharing in delicious And of course thanks for your effort
Fantastic site A lot of helpful info here Im sending it to some buddies ans additionally sharing in delicious And naturally thanks on your sweat
I loved as much as youll receive carried out right here The sketch is tasteful your authored material stylish nonetheless you command get bought an nervousness over that you wish be delivering the following unwell unquestionably come more formerly again since exactly the same nearly a lot often inside case you shield this hike
Your blog is a beacon of light in the often murky waters of online content. Your thoughtful analysis and insightful commentary never fail to leave a lasting impression. Keep up the amazing work!
I loved as much as youll receive carried out right here The sketch is attractive your authored material stylish nonetheless you command get bought an nervousness over that you wish be delivering the following unwell unquestionably come more formerly again as exactly the same nearly a lot often inside case you shield this hike
helloI like your writing very so much proportion we keep up a correspondence extra approximately your post on AOL I need an expert in this space to unravel my problem May be that is you Taking a look forward to see you
Nice blog here Also your site loads up very fast What host are you using Can I get your affiliate link to your host I wish my site loaded up as quickly as yours lol
Fantastic beat I would like to apprentice while you amend your web site how could i subscribe for a blog site The account helped me a acceptable deal I had been a little bit acquainted of this your broadcast offered bright clear concept
My brother suggested I might like this website He was totally right This post actually made my day You cannt imagine just how much time I had spent for this information Thanks
Whoa, that blog style is awesome. For what duration have you been blogging? You made it look so easy. Overall, your website looks great, and the content is much better.
Your writing is like a breath of fresh air in the often stale world of online content. Your unique perspective and engaging style set you apart from the crowd. Thank you for sharing your talents with us.
My brother was absolutely right when he suggested that I would like this website. You have no idea how much time I spent looking for this information, but this post made my day.
Fantastic site Lots of helpful information here I am sending it to some friends ans additionally sharing in delicious And of course thanks for your effort
Thanks I have recently been looking for info about this subject for a while and yours is the greatest I have discovered so far However what in regards to the bottom line Are you certain in regards to the supply
Attractive section of content I just stumbled upon your blog and in accession capital to assert that I get actually enjoyed account your blog posts Anyway I will be subscribing to your augment and even I achievement you access consistently fast
This website is astounding. The radiant substance uncovers the administrator’s excitement. I’m awestruck and envision more such extraordinary presents.
Recently I found this great website, they create engaging content for their audience. The site owner excels at informing customers. I’m excited and hope they keep up their magnificent skills.
I wonder how much work goes into creating a website this excellent and educational. I’ve read a few really good things here, and it’s definitely worth saving for future visits.
Just wish to say your article is as surprising The clearness in your post is just cool and i could assume youre an expert on this subject Fine with your permission allow me to grab your RSS feed to keep updated with forthcoming post Thanks a million and please keep up the enjoyable work
Just wish to say your article is as surprising The clearness in your post is just cool and i could assume youre an expert on this subject Fine with your permission allow me to grab your RSS feed to keep updated with forthcoming post Thanks a million and please keep up the enjoyable work
I loved as much as you will receive carried out right here The sketch is tasteful your authored subject matter stylish nonetheless you command get got an edginess over that you wish be delivering the following unwell unquestionably come further formerly again as exactly the same nearly very often inside case you shield this hike
Magnificent beat I would like to apprentice while you amend your site how can i subscribe for a blog web site The account helped me a acceptable deal I had been a little bit acquainted of this your broadcast offered bright clear idea
I simply had to leave your website before saying how much I appreciated the regular information you provided for your users. I will definitely be back frequently to see what new posts have been made.
I loved as much as you will receive carried out right here The sketch is tasteful your authored subject matter stylish nonetheless you command get got an edginess over that you wish be delivering the following unwell unquestionably come further formerly again as exactly the same nearly very often inside case you shield this hike
Hi i think that i saw you visited my web site thus i came to Return the favore I am attempting to find things to improve my web siteI suppose its ok to use some of your ideas
Hi my loved one I wish to say that this post is amazing nice written and include approximately all vital infos Id like to peer more posts like this
wflmj3loxjv1@gmail.com