Clasificar imágenes es un tema común en redes neuronales. Aquí tienes un ejemplo simple de cómo realizar esta tarea utilizando TensorFlow y la biblioteca Keras, vamos a realizar la clasificación de imágenes de gatos y perros:
import tensorflow as tf
from tensorflow.keras.preprocessing.image import ImageDataGenerator
from tensorflow.keras.models import Sequential
from tensorflow.keras.layers import Conv2D, MaxPooling2D, Flatten, Dense
import matplotlib.pyplot as plt
# Directorios de datos (ajusta las rutas a tu configuración)
train_dir = '/content/entrenamiento/'
test_dir = '/content/prueba/'
# Preprocesamiento de datos
train_datagen = ImageDataGenerator(rescale=1./255)
test_datagen = ImageDataGenerator(rescale=1./255)
train_generator = train_datagen.flow_from_directory(
train_dir,
target_size=(150, 150),
batch_size=20,
class_mode='binary',
classes=['gatos', 'perros']
)
test_generator = test_datagen.flow_from_directory(
test_dir,
target_size=(150, 150),
batch_size=20,
class_mode='binary',
classes=['gatos', 'perros']
)
# Construir el modelo
model = Sequential([
Conv2D(32, (3, 3), activation='relu', input_shape=(150, 150, 3)),
MaxPooling2D(2, 2),
Conv2D(64, (3, 3), activation='relu'),
MaxPooling2D(2, 2),
Conv2D(128, (3, 3), activation='relu'),
MaxPooling2D(2, 2),
Flatten(),
Dense(512, activation='relu'),
Dense(1, activation='sigmoid')
])
# Compilar el modelo
model.compile(loss='binary_crossentropy',
optimizer='adam',
metrics=['accuracy'])
# Entrenar el modelo
history = model.fit(train_generator, epochs=15, validation_data=test_generator)
# Evaluar el modelo
test_loss, test_acc = model.evaluate(test_generator)
print(f'Precisión en el conjunto de prueba: {test_acc * 100:.2f}%')
# Visualizar imágenes y sus predicciones
sample_images, sample_labels = next(test_generator)
predictions = model.predict(sample_images)
for i in range(len(sample_labels)):
label = 'Gato' if sample_labels[i] == 0 else 'Perro'
prediction = 'Gato' if predictions[i] < 0.5 else 'Perro'
plt.imshow(sample_images[i])
plt.title(f'Clase real: {label}\nPredicción: {prediction}')
plt.show()
# Visualizar la pérdida y la precisión durante el entrenamiento
plt.plot(history.history['accuracy'], label='Precisión de entrenamiento')
plt.plot(history.history['val_accuracy'], label='Precisión de prueba')
plt.xlabel('Épocas')
plt.ylabel('Precisión')
plt.legend(loc='lower right')
plt.show()
- Importación de Bibliotecas: Se importan las bibliotecas necesarias, incluyendo TensorFlow (para el aprendizaje profundo), ImageDataGenerator (para el preprocesamiento de imágenes), Sequential (para la creación del modelo), Conv2D y MaxPooling2D (capas convolucionales), Flatten y Dense (capas completamente conectadas) y Matplotlib (para la visualización).
- Directorios de Datos: Se definen las rutas a los directorios que contienen los datos de entrenamiento y prueba. Asegúrate de ajustar estas rutas a tu propia configuración, de modo que los directorios de datos estén correctamente especificados, deben contener los directorios gatos y perros en los directorios de prueba y entrenamiento.
- Preprocesamiento de Datos: Se configuran generadores de datos para preprocesar las imágenes. El
ImageDataGenerator
se utiliza para escalar los valores de píxeles en el rango [0, 1] al dividirlos por 255. Esto normaliza las imágenes. - Generadores de Datos de Entrenamiento y Prueba: Se utilizan los generadores de datos para cargar las imágenes desde los directorios de datos de entrenamiento y prueba. Además, se especifica que hay dos clases: «gatos» y «perros».
- Construcción del Modelo: Se crea un modelo de red neuronal secuencial utilizando Keras. El modelo consiste en capas convolucionales y capas completamente conectadas. El modelo se define para procesar imágenes de 150×150 píxeles con 3 canales de color (RGB).
- Compilación del Modelo: El modelo se compila especificando la función de pérdida (‘binary_crossentropy’ para clasificación binaria), el optimizador (‘adam’) y las métricas a seguir (‘accuracy’ para precisión).
- Entrenamiento del Modelo: El modelo se entrena con los datos de entrenamiento utilizando el generador de datos de entrenamiento. Se ejecutan 15 épocas y se utiliza el conjunto de prueba para la validación. El historial del entrenamiento se almacena en
history
. - Evaluación del Modelo: Se evalúa el modelo en el conjunto de prueba para calcular la precisión en los datos de prueba.
- Visualización de Imágenes y Predicciones: Se seleccionan un conjunto de imágenes de prueba y se realizan predicciones en ellas. Luego, se muestra cada imagen junto con su etiqueta real y la etiqueta predicha por el modelo.
- Visualización de la Pérdida y la Precisión: Se visualiza cómo cambia la precisión en el conjunto de entrenamiento y prueba a lo largo de las épocas.
Precisión en el conjunto de prueba: 100.00%
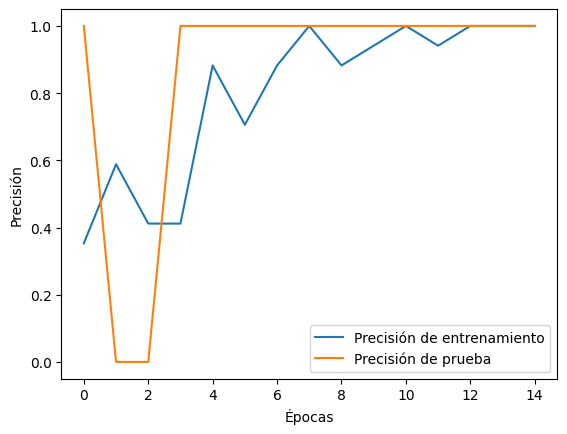
My brother suggested I might like this blog He was totally right This post actually made my day You can not imagine simply how much time I had spent for this info Thanks
Lois Sasson very informative articles or reviews at this time.
Thinker Pedia For the reason that the admin of this site is working, no uncertainty very quickly it will be renowned, due to its quality contents.
Fantastic site A lot of helpful info here Im sending it to some buddies ans additionally sharing in delicious And naturally thanks on your sweat
Fantastic beat I would like to apprentice while you amend your web site how could i subscribe for a blog site The account helped me a acceptable deal I had been a little bit acquainted of this your broadcast offered bright clear concept
Fran Candelera Nice post. I learn something totally new and challenging on websites
GlobalBllog This is my first time pay a quick visit at here and i am really happy to read everthing at one place
GlobalBllog I like the efforts you have put in this, regards for all the great content.
Insanont This is really interesting, You’re a very skilled blogger. I’ve joined your feed and look forward to seeking more of your magnificent post. Also, I’ve shared your site in my social networks!
Mating Press You’re so awesome! I don’t believe I have read a single thing like that before. So great to find someone with some original thoughts on this topic. Really.. thank you for starting this up. This website is something that is needed on the internet, someone with a little originality!
Family Dollar I truly appreciate your technique of writing a blog. I added it to my bookmark site list and will
I do trust all the ideas youve presented in your post They are really convincing and will definitely work Nonetheless the posts are too short for newbies May just you please lengthen them a bit from next time Thank you for the post
allegheny county real estate This is really interesting, You’re a very skilled blogger. I’ve joined your feed and look forward to seeking more of your magnificent post. Also, I’ve shared your site in my social networks!
Simplywall I very delighted to find this internet site on bing, just what I was searching for as well saved to fav
Bạn thật tài năng! Bài viết này thật sự rất ấn tượng. Tôi đã học được rất nhiều điều mới từ những chia sẻ của bạn. Tôi sẽ chia sẻ bài viết này với bạn bè của mình.
Stunning work! This article is a masterclass in conveying information in a compelling narrative.
Real Estate naturally like your web site however you need to take a look at the spelling on several of your posts. A number of them are rife with spelling problems and I find it very bothersome to tell the truth on the other hand I will surely come again again.
Real Estate Great information shared.. really enjoyed reading this post thank you author for sharing this post .. appreciated
I just could not depart your web site prior to suggesting that I really loved the usual info an individual supply in your visitors Is gonna be back regularly to check up on new posts
Program iz very informative articles or reviews at this time.
Business dicker I appreciate you sharing this blog post. Thanks Again. Cool.
Temp mail I like the efforts you have put in this, regards for all the great content.
Fantastic site Lots of helpful information here I am sending it to some friends ans additionally sharing in delicious And of course thanks for your effort
I do trust all the ideas youve presented in your post They are really convincing and will definitely work Nonetheless the posts are too short for newbies May just you please lengthen them a bit from next time Thank you for the post
Temp mail I like the efforts you have put in this, regards for all the great content.
of course like your website but you have to check the spelling on several of your posts A number of them are rife with spelling issues and I in finding it very troublesome to inform the reality on the other hand I will certainly come back again
Usually I do not read article on blogs however I would like to say that this writeup very compelled me to take a look at and do so Your writing taste has been amazed me Thanks quite nice post
Nice blog here Also your site loads up very fast What host are you using Can I get your affiliate link to your host I wish my site loaded up as quickly as yours lol
What i do not realize is in fact how you are no longer actually much more wellfavored than you might be right now Youre very intelligent You recognize thus considerably in relation to this topic made me in my view believe it from numerous numerous angles Its like men and women are not fascinated until it is one thing to do with Lady gaga Your own stuffs excellent All the time handle it up
you are truly a just right webmaster The site loading speed is incredible It kind of feels that youre doing any distinctive trick In addition The contents are masterwork you have done a great activity in this matter
I loved as much as youll receive carried out right here The sketch is attractive your authored material stylish nonetheless you command get bought an nervousness over that you wish be delivering the following unwell unquestionably come more formerly again as exactly the same nearly a lot often inside case you shield this hike
My brother suggested I might like this website He was totally right This post actually made my day You cannt imagine just how much time I had spent for this information Thanks
I was suggested this web site by my cousin Im not sure whether this post is written by him as no one else know such detailed about my trouble You are incredible Thanks
What i dont understood is in reality how youre now not really a lot more smartlyfavored than you might be now Youre very intelligent You understand therefore significantly in terms of this topic produced me personally believe it from a lot of numerous angles Its like women and men are not interested except it is one thing to accomplish with Woman gaga Your own stuffs outstanding Always care for it up
helloI like your writing very so much proportion we keep up a correspondence extra approximately your post on AOL I need an expert in this space to unravel my problem May be that is you Taking a look forward to see you
My brother recommended I might like this web site He was totally right This post actually made my day You cannt imagine just how much time I had spent for this information Thanks
This was beautiful Admin. Thank you for your reflections.
This is my first time pay a quick visit at here and i am really happy to read everthing at one place